Share your science:
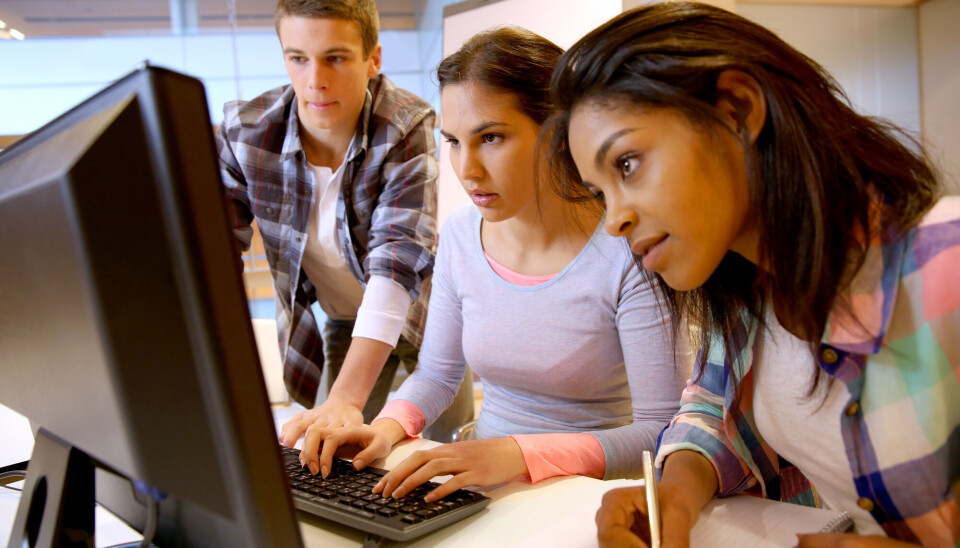
The future of artificial intelligence in education
SHARE YOUR SCIENCE: Using ChatGPT, the students reports surpassed the quality of many research papers. How can subject matter experts stay relevant for software engineers?
In the rapidly evolving world of technology, my research over the last decade has been guided by the question of how we can harness technology, using it mindfully, to catalyze societal benefits.
Amidst complex challenges like preserving individual privacy and resisting corporate pressures, I have found my own research affected. Categorizing technology as good or bad does not provide much insight, but rather in examining and refining our relationship to it, focusing on its judicious use, we can, at least to some degree, have an informed discussion of its meaning to us.
Reliable, ethical, and secure
My current research focuses on Trustworthy AI (TAI) practices. TAI is a concept in AI research where the goal is to create AI systems that operate in a reliable, ethical, and secure manner. Through a large multi-disciplinary network, called the Z-Inspection® initiative, we have been involved in several European cases, assessing AI use in settings ranging from healthcare to government.
When assessing an AI system, our initial question concerns the intended purpose. It might seem simple to answer, but considering the TAI principles, it is not. Product owners often struggle to articulate their system's organizational use, making the task far more complex than it appears at first glance.
Reflecting on models
The integration of foundation models like ChatGPT into education is not a binary decision. Foundation models are the latest generation of AI, capable of reasoning based on interactive input, a trait often ascribed to humans.
In collaboration with Lester Lasrado, who heads the Information Systems Master's program, we agreed that students studying Big Data Analytics should also learn about foundation models. They must learn to reflect on how these models work and understand their limitations.
In the course, we made it clear to students that they could use ChatGPT and lectured on how language models function. We emphasized that students must learn the limitations of the AI and take personal responsibility for the tool's output. We also emphasized that directly copying/pasting from ChatGPT is not ok.
Surpassed the quality of many research papers
Having taught the course several years before, without ChatGPT, I had a sense of what to expect regarding student output. The introduction of ChatGPT to the course resulted, however, in some surprising outcomes.
It helped students with various tasks such as debugging, code generation, reasoning about problems and their sub-components, advising on research questions, designing and refining experiments, and report writing. The outcome was that students' work was so comprehensive that grading sometimes became a challenge. Some reports surpassed the quality of many research papers I've reviewed for conferences.
A happy student is a learning student, after all!
The introduction of this tool also had an unexpected benefit, it shifted the learning dynamic. Complaints from students who knew the subject theory but struggled with coding decreased. The usual student frustration, often stemming from an inability to express themselves in code, was largely absent. A happy student is a learning student, after all!
How to stay relevant
Deciding how to approach AI in education presents a long-term complex problem. As coding becomes much more accessible and “cheaper”, any discipline that collects and relies on data must equip students for quantitative experimentation, including specifying questions and requirements for implementing code to process data.
The division of work between subject matter experts and software engineers will most certainly change as a result. To stay relevant in a future post-AI job market, subject matter experts must understand how to implement their own experiments using AI tools.
Not at all much different from the use of text and spreadsheet editors today. However, the quantitative analysis performed will demand much more than elementary statistical methods and should include probabilistic ML methods to process data.
Lecturers must be supported
To address this, lecturers must be supported in learning how to deal with AI within their different areas of expertise. While some areas have a more natural progression toward refining data into knowledge, many will need educational support.
The challenge this time is that we cannot look toward the industry to understand the application and then devise a curriculum to prepare students, but rather that we may need to address the fundamental didactical reasoning in how we construct our pedagogy.
Share your science or have an opinion in the Researchers' zone
The ScienceNorway Researchers' zone consists of opinions, blogs and popular science pieces written by researchers and scientists from or based in Norway.
Want to contribute? Send us an email!